Overview
Research in our group uses theoretical approaches to gain insight into cellular mechanisms in vivo. Our main interests focus on development and regeneration, processes driven by diverse types of stem cells. These stem cell systems nevertheless share essential characteristics, such as the ability to specify and maintain cell fates in dynamic and uncertain environments. We address questions of cell dynamics, signaling, and fate control by mathematical modeling, statistical inference, computational method development, and data analysis. Below are examples of current research directions.
Single-cell analysis of cell state transitions
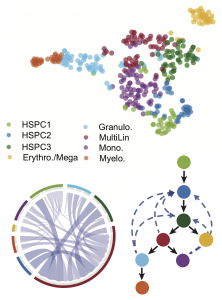
Top: Hematopoietic cell states identified by SoptSC (data from here). Bottom: cell-cell signaling interaction network (mediated by Tgf-β) and lineage relationships define cell state transitions during hematopoiesis.
Information about the signals that are passed between cells (via cell-cell communication) can be directly inferred from data, using e.g. expression of paracrine signaling factors, or can be inferred from models that explore different possible topologies of coupled gene regulation and cell-cell communication networks. By the integration of data with dynamical systems models, it becomes possible to perform parameter inference and model selection at the level of single cells.
Modeling stem cell lineage and signaling dynamics
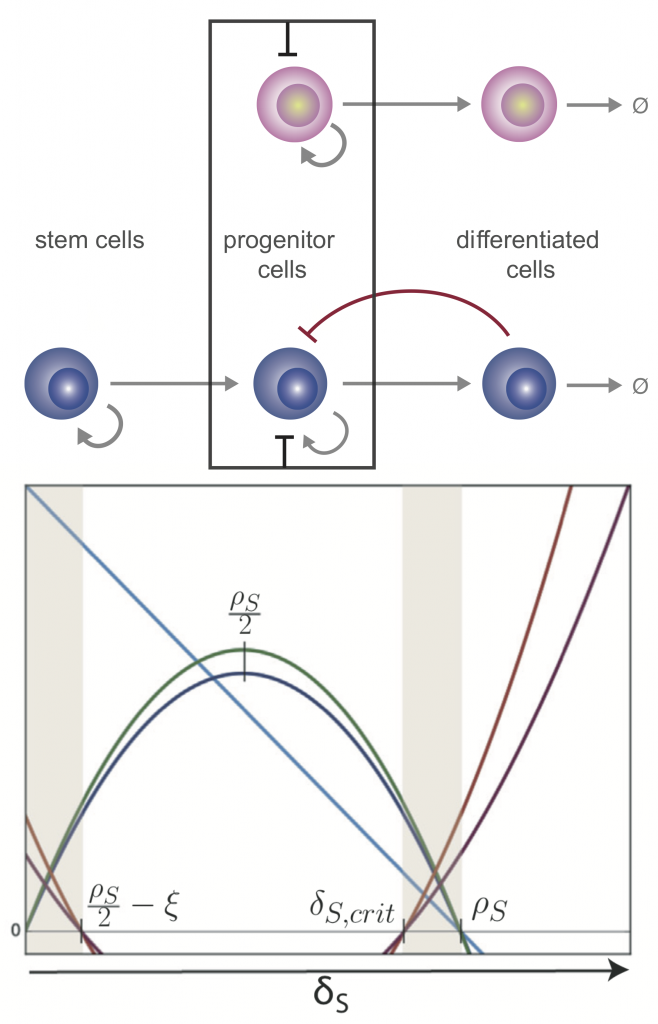
Top: competitive dynamics of a stem cell lineage model with progeny- and niche-mediated feedback. Bottom: Analysis of the critical points determines regions (shaded) where healthy and leukemia stem cells coexist, and prognosis is most uncertain.
We perform parameter inference and model selection within a Bayesian framework to appropriately account for our knowledge and uncertainties about these systems, and to be able to predict cell dynamics under new perturbations. Analysis of mechanistic models, in light of data, gives insight into the tightly regulated transcriptional programs that underlie stem cell phenotype. For example, we can investigate how a balance of cell division, differentiation, and migration controls tissue development and regeneration.
Multiscale tissue dynamics
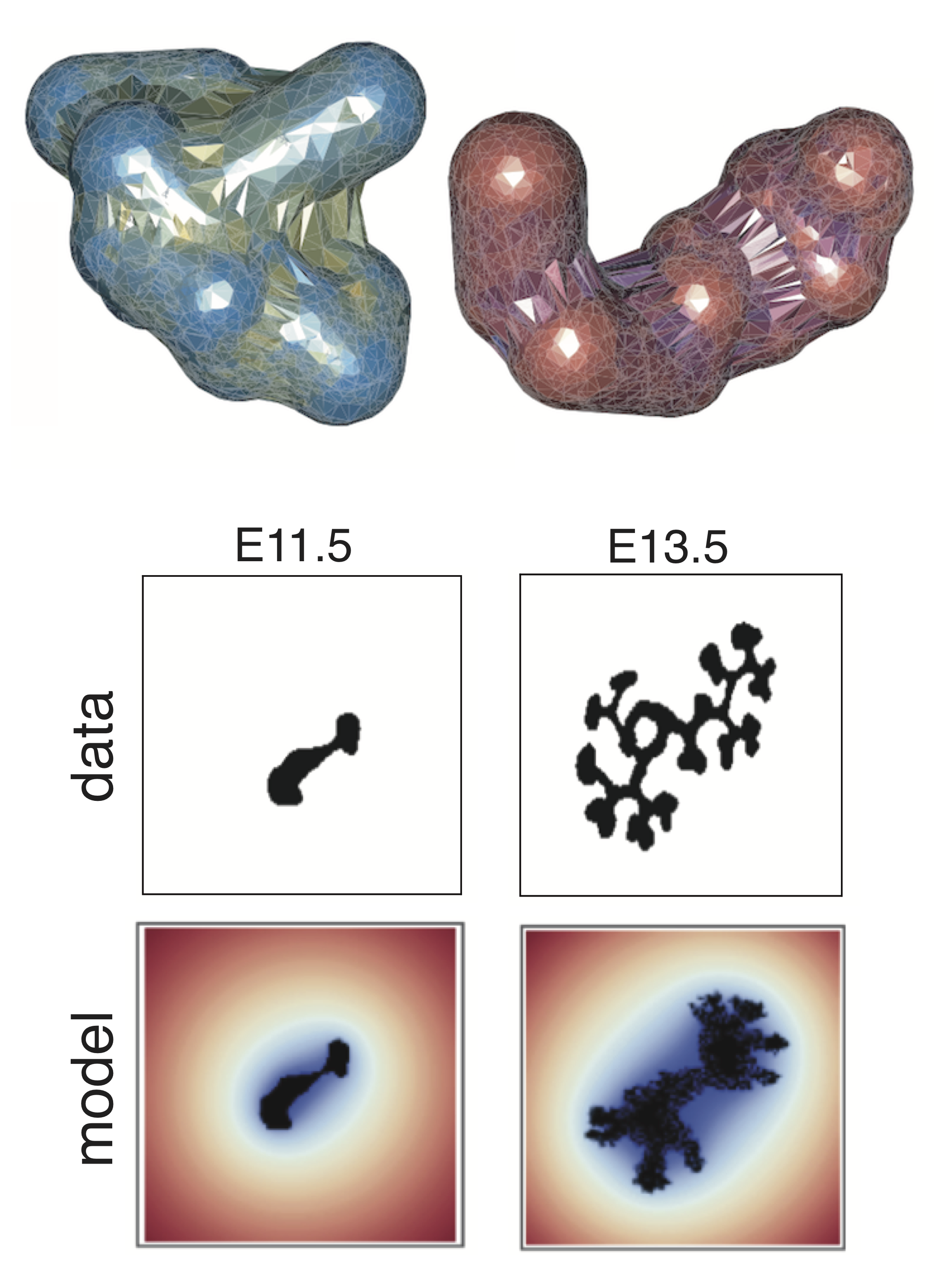
We develop individual cell-based models that target specific biological questions. For example, we seek to understand how stem cells interact with niches in 3D space, to predict what factors are crucial to initiate epithelial tissue branching, and to study interactions between the immune system and cancer. These models often contain hybrid components, for example continuous components (PDEs) to describe diffuse factors such as morphogens, and agent-based on/off lattice components to describe the agents (typically biological cells) that interact and are regulated both intrinsically and by the surrounding microenvironment.
Some examples of such multiscale models include kidney branching organogenesis and inflammation-induced tumorigenesis. Simulation times can become prohibitive for high-throughput analyses (e.g. parameter inference) of such models, and so we work on computational methods to improve simulation efficiency and to improve/facilitate Bayesian parameter inference. The overarching goal for each of these models is to be able to predict the emergent tissue-level behavior resulting from dynamic and noisy cell and molecular interaction networks.